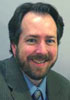
Summary Bullets:
- In our rush to capitalize upon big data, we have come a long way in ensuring that our decisions are based upon the most recent, complete and trustworthy data.
- However, we have not yet applied those lessons to the human heart, which must suffer the slings and arrows of cognitive bias.
I think it’s safe to say that we have achieved a workable level of data democratization within the enterprise wherein business stakeholders, not just data scientists, can access and act upon data. When lightweight data discovery and visualization tools entered the scene a few years ago and began breaking down the barriers between those two constituencies, things looked a bit treacherous. How could we be sure everyday business users had the right data, selected the right chart or drew the right conclusion? Was it really a good idea to let ‘Joe User’ meld data from a personal spreadsheet with information that has been carefully curated within a data warehouse?
We have come a long way since then in ensuring that our decisions are based upon the most recent, complete and trustworthy data. Most BI and data discovery and visualization tools now come equipped with a raft of safety measures such as guided workflows informed by cognitive tools and machine learning. These serve as a safety net of sorts, preventing users from basing decisions upon unsound data or ill-advised questions. Let’s call that data quality. But, what about decision quality? What about the decisions we make based upon quality data?
As much as I admire many of the cognitive tools we have at our disposal right now – tools like IBM’s Watson Analytics, which can quite reliably score the quality of a data set and quite accurately suggest the best questions to ask of that data – those tools cannot rule out the many cognitive biases we mere humans apply to the decision making process. Take for example the biggest elephant in the room, confirmation bias (or its counterpart, belief bias). It is impossible to ask a question without first knowing the answer or at least the expected answer. What if the results don’t match that answer? Unfortunately, and often unconsciously, we seek to find a reason for and a resolution of the discrepancy to make the facts match our expectations. That’s just one example. How about selection bias, where what we choose to look at greatly impacts the final outcome, or the framing effect, conjunction fallacies, decoy effect, et al.?
What we need perhaps is to operationalize the conclusions we draw from data, to push our assessment of a given response back into the system itself where we can then apply our cognitive, machine learning and other technological (read unbiased) heuristic trickery to the task of ferreting out human bias. But, can we really take humans out of the decision chain and leave important decisions to machines, which cannot by their very nature fall prey to undetectable errors such as a confirmation bias?
As much as I believe in heuristics (we taught a machine to play Go, didn’t we?), I think at the end of the day we’ll need a human solution to this very human problem. We should encourage businesses to employ mitigation strategies such as building a detailed decision tree (“how did you come to that conclusion?”). But, most importantly, we should teach them how to identify and counter fallacies in reasoning. Simply teaching users how the context in which they frame a question influences the perceived answer can go a long way. Regardless, the bottom line is simply this: People are just as important if not more so than the data they interpret. And we must invest in them as much as in the data if we are truly to reap the benefits of analytics.